Sheil Research Group
Our research prioritises digital engineering as a key enabler of the Laing O’Rourke Centre for Construction Engineering & Technology mission to transform construction. Specifically, our work focuses on transforming the productivity and sustainability of underground construction operations. This has included the use of probabilistic machine learning, underground digital twins, ground modelling, physics-informed neural networks for knowledge discovery, live construction monitoring and fundamental soil-structure mechanics. Applications include, but are not limited to, tunnelling, basement construction, shafts, deep excavations, mining, and deep foundations.
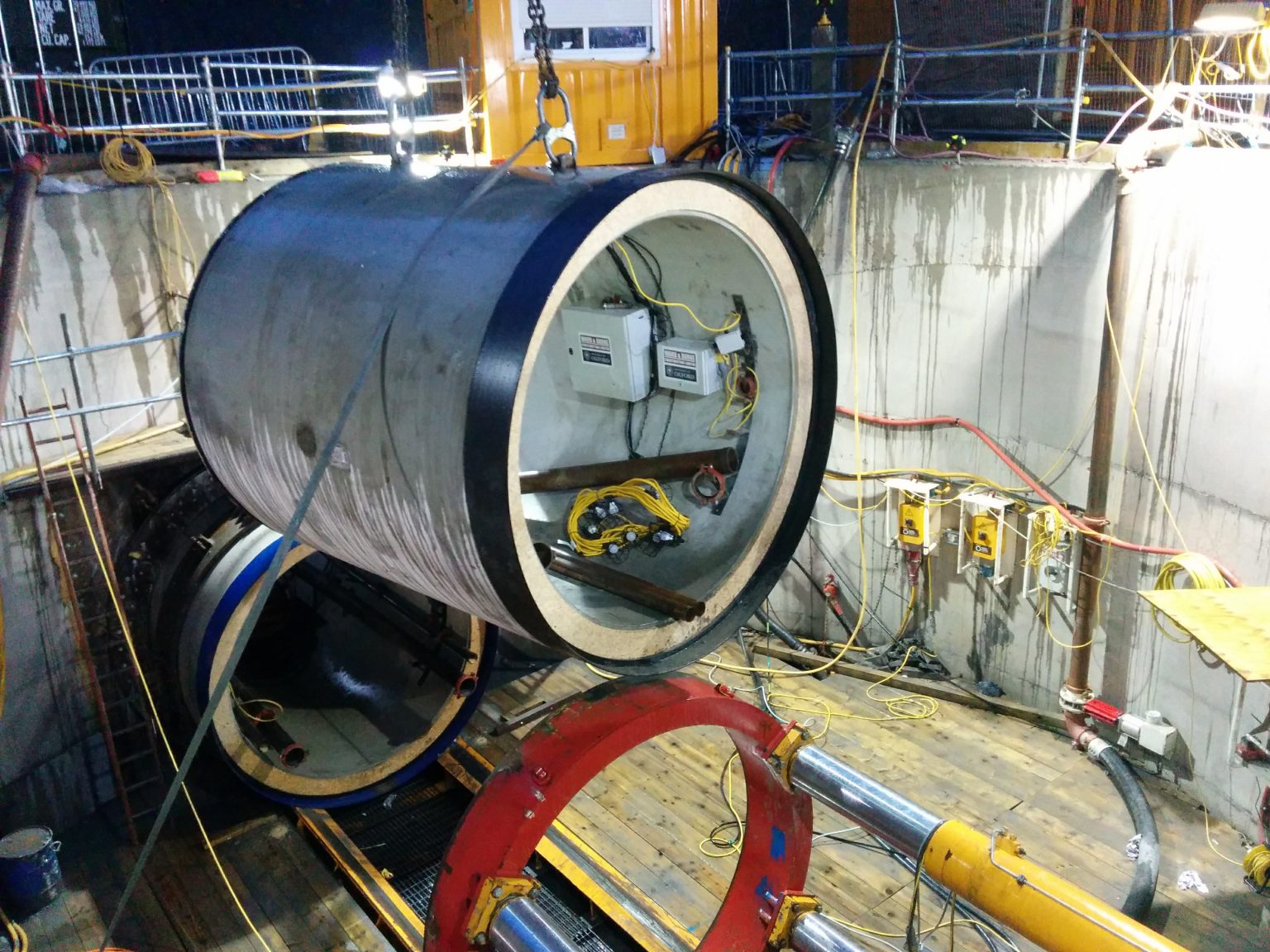
Digital Engineering
We combine machine learning with underground digital twins to inform construction operations
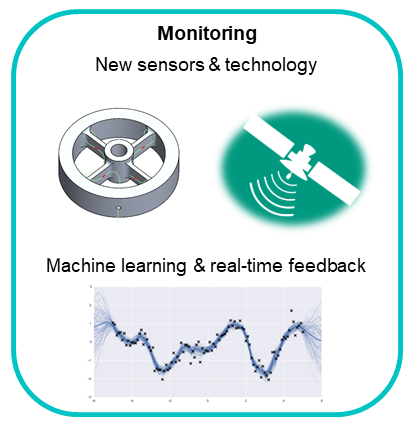
Live Monitoring
We develop new sensors and live monitoring techniques for underground construction
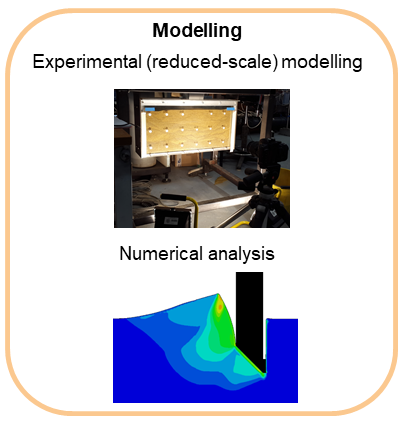
Modelling
We perform bespoke laboratory experiments and advanced numerical analyses to achieve new insights into soil-structure interaction during underground construction
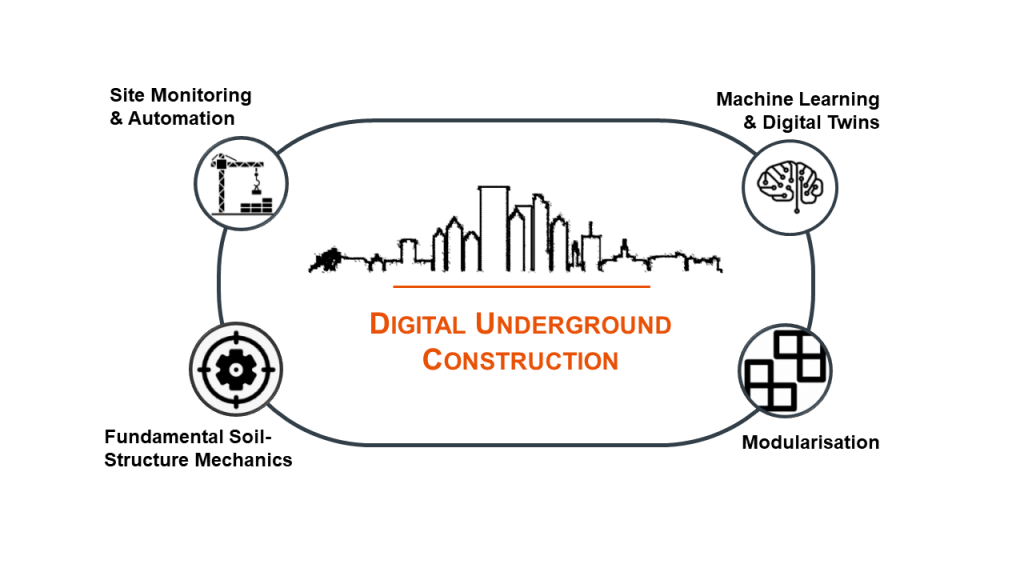
- Bayesian machine learning for construction operations
- Underground digital twins
- Live construction monitoring
- Novel sensor design & development
- Physics-informed neural networks for knowledge discovery
- Soil-structure mechanics
- Construction support fluids
Cutting Edge Science for Industry Implementation
Our research is informed by and tailored for real-world implementation. We value industry input and collaboration in any form. Do not hesitate to get in touch to find out more about how we can help overcome practical pain points.
Current Research Projects
Digital basements
Student: Currently recruiting
Advanced 3D modelling and live site monitoring are common for urban basement construction. However, there is usually no interaction between these activities and the lack of feedback from monitored behaviour currently inhibits design and construction optimisation. More importantly, as the industry is forced to reuse old inner-city foundations, monitored behaviour will be become critical: (a) to make a convincing case for reuse and (b) to inform the design of the new superstructure. For the first time, this project will develop digital twins for basements to enable design and construction optimisation. The project will develop autonomous connections between 3D design models, structural (e.g. finite element) models and state-of-the-art real-time site monitoring. The monitored data will feed back into the design process ‘on-the-fly’ and life-time monitored behaviours will be stored in the digital twin to inform future foundation reuse. The workflow will be validated on a candidate construction project in the UK.
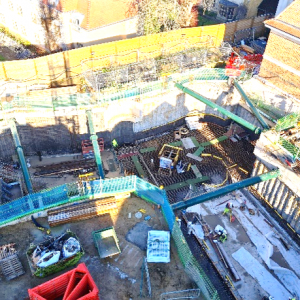
Digital twins for as-built shield tunnels
Student: Mr Wei Lin
Deformation and defects in as-built shield tunnels present significant challenges for tunnel managers and engineers, particularly in urban environments. Determining the current mechanical status (e.g. stress level) of shield tunnels becomes critical to guide repair and reinforcement strategies. However, existing evaluation and analysis methods either lack precision or are prohibitively costly. The development of BIM and AI have brought promising opportunities for automatic and digital evaluation and analysis of existing tunnels. The objective of this work is to achieve automatic mechanical evaluation and analysis of as-built shield tunnels using digital twins by combining laser scanning, AI algorithms, BIM and finite element analysis.
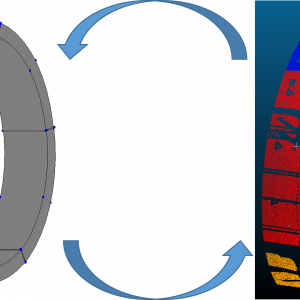
Green underground construction
Student: Mr Alexander Swallow
To keep pace with growing populations and to account for deteriorating infrastructure, a substantial amount of new infrastructure is required in both the UK and worldwide. However, the construction sector is one of the biggest consumers of resources and raw materials worldwide and is responsible for 39% of all carbon emissions. If the UK is to meet its target of net zero emissions by 2050, then significant step-changes in how we construct our infrastructure are required. This research will deliver the underpinning engineering science for next-generation construction operations optimised, for the first time, to achieve near-zero carbon emissions. This will be achieved through the use of a combination of material science, reduced-scale laboratory modelling, monitoring of live construction sites and advanced AI optimisation techniques.
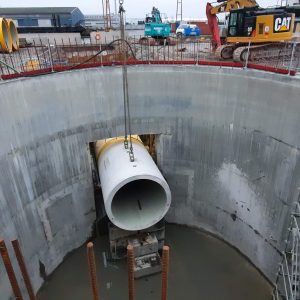
Monitoring the construction of deep bunkers for energy-from-waste facilities
Student: Mr Pete Hensman
Industry sponsor: Ward and Burke Construction Ltd
This research aims to improve understanding of soil-structure interaction applied to deep bunkers for the construction of energy-from-waste facilities. This will be achieved through the use of real-time site monitoring and complementary finite element modelling. Data acquired from the field monitoring will be used to calibrate and verify the developed numerical models, whilst also creating a database for the development of simplified empirical methods. This field data can also be used to develop a system which provides real time feedback to site personnel, actively informing the construction process, and promoting a proactive approach to risk management and mitigation.
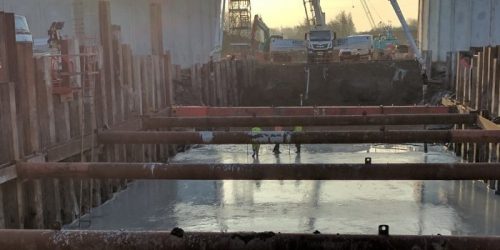
Fusing computer vision with AI for structural health monitoring
Student: Ms Jiaxu Zuo
Primary supervisor: Professor Sinan Acikgoz
This project will explore how to improve digital image correlation measurements to cope with lighting changes and camera movements using machine learning techniques. The improved algorithms for displacement and strain measurements will enable enhanced long-term structural monitoring of assets and material identification of existing assets.
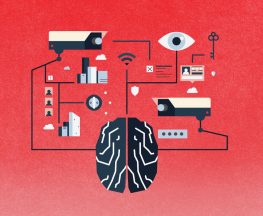
Design & performance of pipe-jacked tunnel drives
Student: Mr Bryn Phillips
Industry sponsor: Ward and Burke Construction Ltd
Microtunnelling is an increasingly popular means of trenchless installation for tunnels of between 600 mm and 3500 mm internal diameter and drive lengths of less than 1500m (typically). One of the greatest risks in long-drive microtunnelling is the development of excessive soil-structure penetration resistances, to the point where they exceed the total available jacking thrust. This project seeks to address this shortcoming through the development and monitoring of instrumented ‘smart’ tunnel pipes as well as bespoke reduced-scale tunnel-soil interface testing in the laboratory.
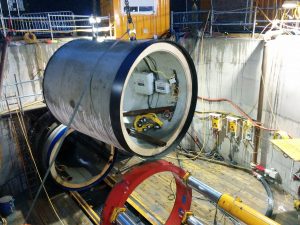
Modelling soil-structure interaction for large-diameter shafts
Student: Mr Jack Templeman
Sponsor: EPSRC
An increasingly common method of shaft construction is open-dug caisson sinking. This is a type of top-down construction in which a reinforced concrete wall is cast at the surface and progressively sunk into the ground. The sinking phase presents a number of challenges during construction including maintaining caisson verticality, controlling the rate of sinking and minimizing soil resistance through the use of lubricating fluids. This research aims to advance current understanding of soil-structure interaction for large-diameter caisson shaft construction. This will be achieved using large-deformation numerical modelling and complementary laboratory testing.
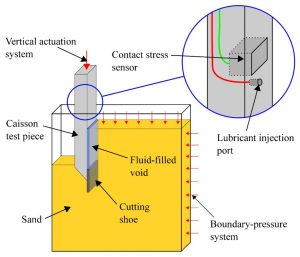
Satellite-enabled early warning system for geotechnical structures
Student: Ms Maral Bayaraa
Sponsor: Royal Commission for the Exhibition of 1851
Industry sponsor: Satellite Applications Catapult
From hundreds of km in space, satellite-Interferometric Synthetic-Aperture Radar (InSAR) analysis allow detection of ground motion to a high level of precision. However, complexities in converting the raw data to actionable information has so far limited authorities’ ability to practically monitor these structures from space. To help solve this, this research is creating a framework for geotechnical validation of the satellite measurements by developing 3D numerical models capable of simulating the underpinning mechanics of the observed soil movements. Deep learning experiments will be used to create an end-to-end scalable early warning tool, tested on historical failures and other geotechnical applications. The research will be focused on tailings dams and deep excavations.
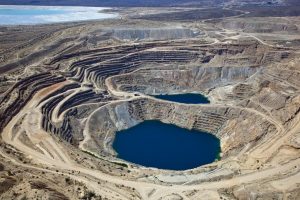
Damage detection for critical infrastructure using computer vision & deep learning
Student: Yixiong Jing
PI: Professor Sinan Acikgoz
Ageing infrastructure places a higher demand on both the frequency and scale of inspections. These inspections are time-consuming, costly and are prone to human error. This project seeks to develop an automated, flexible, accurate and efficient process to identify existing damage in critical infrastructure. This will be achieved using a combination of state-of the art computer vision and deep learning techniques.
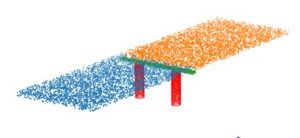
Behaviour of construction support fluids
Students: Mr Daniel McNamara, Mr Bryn Phillips, Ms Alicia Leong, Mr Diarmid Xu
Sponsors: Ward and Burke Construction Ltd (bentonite support fluids), EPSRC and Arup (polymer support fluids)
Bentonite- and polymer-based support fluids are widely used in the construction industry to provide temporary hydraulic support to unstable ground, for example in tunnelling works, caisson sinking and deep excavations. Whilst most civil engineering designs must account for the performance of support fluids during construction, they often do so in an ad hoc manner, relying primarily on the development of empiricism from accumulated field experience. This research will result in new design rules and testing techniques for construction support fluids, with significant benefits for the construction industry in terms of risk, material use, and time to construct. These objectives will be achieved through fieldwork, laboratory testing and theoretical analysis.
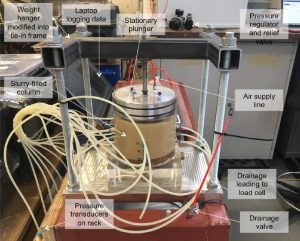
Design method updating using Bayesian machine learning
Sponsor: Royal Academy of Engineering, EPSRC
While significant advances have been made in prediction modelling, uncertainty surrounding the assessment of geotechnical parameters and underground conditions (e.g. karst caverns, faults,coal veins) remains the main barrier to accurate prediction of construction behaviour. Back-analysis of soil parameters within an observational framework has been applied widely for geotechnical applications such as slope stability, pile capacity and braced excavations. This study presents a Bayesian approach to update uncertain model parameters to inform rational strategies for optimising construction operations using the latest monitoring data acquired during the project. The proposed framework is applied to pipe jacking and caisson construction.
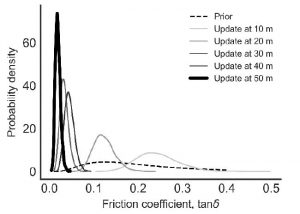
Anomaly detection for microtunnelling
Sponsor: Royal Academy of Engineering, EPSRC
The proliferation of data collected by modern tunnel boring machines presents a substantial opportunity for the application of data-driven anomaly detection (AD) techniques that can adapt dynamically to site specific conditions. Based on jacking forces measured during microtunnelling, this research explores the potential for AD methods to provide more accurate and robust detection of incipient faults. A selection of the most popular AD methods proposed in the literature, comprising both clustering- and regression-based techniques, are considered.
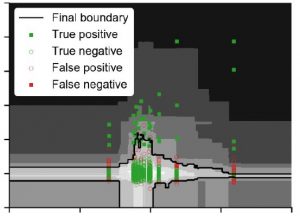
3D ground modelling for tunnelling using probabilistic machine learning
Collaborator: Dr Stephen Suryasentana (University of Strathclyde)
An effective site investigation (SI) campaign is critical for establishing the ground parameters for tunnel design. Optimal SI planning and interpretation is therefore an important element for the future progress of cost-effective underground infrastructure. However, choosing the appropriate SI campaign (number of tests, test locations etc.) to minimise ground-related risk and uncertainty is challenging, as there is a lack of guidance in the design codes for SI planning. Therefore, the SI campaign is usually planned heuristically using ad-hoc engineering judgment. Given the large-scale nature of SI campaigns for some underground infrastructure projects, such as HS2 and the Thames Tideway Tunnel, considerable savings can be realised if a more optimal, automated and adaptive approach is adopted. This project will address these limitations through the integration of state-of-the-art probabilistic machine learning (ML) into the planning process of SI campaigns for tunnelling.
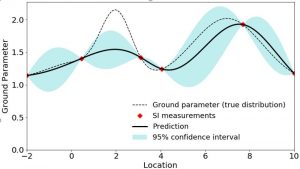
Laboratory testing of construction-induced settlements surrounding caisson shafts
Student: Mr Matthew Willoughby
There is very little guidance in the published literature relating to the soil settlements induced by the construction of large-diameter caisson shafts. This is particularly problematic if these shafts are to be located in close proximity to existing infrastructure. This research involves the development of a new apparatus to investigate the settlement induced by the construction of large-diameter caisson shafts at model scale. Construction-induced soil settlements are measured using soil imaging techniques. The laboratory measurements are being used to inform the development of new empirical design rules and numerical models
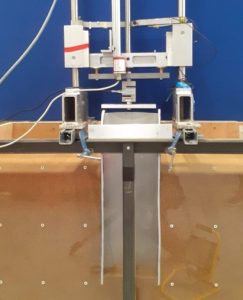
Numerical modelling of soil-structure interaction for laterally-loaded pile groups
Student: Mr Michael Watford
Pile foundations are frequently adopted to support structures founded in areas with unfavourable ground conditions and are often the only feasible solution for supporting large structures such as high-rise buildings, bridge piers and wind turbines. These foundations are typically required to resist significant lateral loads from wind, wave or current actions on the superstructure and/or the foundation itself. Accurate estimation of the role of group effects on the ‘deep-condition’ soil limiting pressure is essential for future development of more rigorous design solutions for laterally loaded pile groups. However, previous work has mainly focused on very small groups (< 5 piles). This work addresses this shortcoming using two−dimensional finite element modelling to assess the influence of group shape and size on the behaviour of laterally loaded pile groups. Additional parameters considered in the modelling include pile spacing and pile-soil interface roughness.
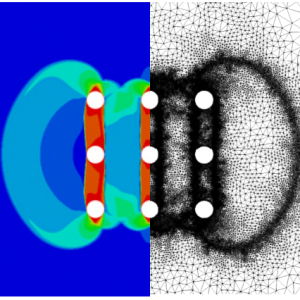
Design & development of smart fibre optic force sensors
Students: Ms Irinka Lamiquiz-Pratt, Mr Jack Templeman
Sponsor: Royal Academy of Engineering
Existing sensor designs use increasingly more complex structures and strain sensing arrangements to measure specific force combinations (e.g. axial force, shear force) independent of temperature effects. This increases sensor cost as well as manufacture and calibration time. This work aims to deliver a step change in force sensor design, manufacture, and operation by fusing state-of-the art fibre optic strain sensing with artificial intelligence techniques.
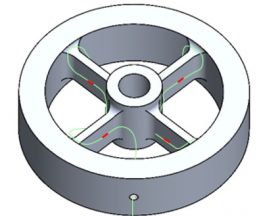